A twisted project that tried to predict criminals from a photo has come to an end
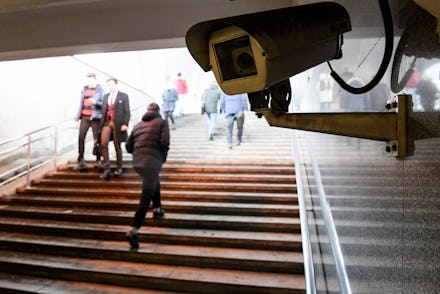
Weeks before Minneapolis police killed George Floyd and the social and political upheaval that followed, researchers at Harrisburg University announced what they viewed as a technological breakthrough. Two professors, along with a former NYPD officer and current PhD student, developed facial recognition software that they claimed could predict the likelihood that a person would commit a crime based solely on a picture of their face. A scientific paper detailing the research was expected to be published later this year, but new calls for police accountability and ongoing concerns about AI systems perpetuating and automating racial biases have stopped the project in its tracks.
The Harrisburg University research project was first made public in May, when the University published a press release announcing the efforts. In it, the researchers are described as having developed "automated computer facial recognition software" that can predict the likelihood a person is going to commit a crime with "80 percent accuracy and with no racial bias." Researchers said the software was "intended to help law enforcement prevent crime." The University also announced that the paper would be published by Springer, the largest publisher of academic texts in the world, in a book titled "Springer Nature – Research Book Series: Transactions on Computational Science & Computational Intelligence.”
Before the paper was published, the very idea of the project was widely panned by experts who viewed the claims as ludicrous. "The idea that a computer model can predict criminality from a photograph is so flawed that I don’t know where to begin, Andrew Guthrie Ferguson, Professor of Law at American University Washington College of Law, tells Mic. "What is the point? Are you going to have police interact with people because of suspicions based on their photograph? If not, what is the purpose of the sorting?"
Within 24 hours, Harrisburg University deleted the press release and distanced itself from the research. In a statement to Mic, a spokesperson for Harrisburg University said that the press release was "removed from the website at the request of the faculty involved in the research." The spokesperson also stated that the University "supports the right and responsibility of university faculty to conduct research and engage in intellectual discourse, including those ideas that can be viewed from different ethical perspectives," but noted, "All research conducted at the University does not necessarily reflect the views and goals of this University."
"It’s just stupid to think that a photograph or any biometric can predict future actions" - Andrew Guthrie Ferguson
Despite the controversy surrounding the research, and the apparent request of even those involved in the project to take down the press release, the paper detailing the facial recognition software was still set to be published by Springer later this year. The Coalition for Critical Technology, a collection of scientists, researchers, and ethicists, decided to intervene. The group published an open letter to Springer urging the company not to publish the research for fear that it would only serve to advance dangerous uses of similar technologies. The group claimed that the ability to predict criminality is "based on unsound scientific premises, research, and methods." Springer responded to the outcry by informing MIT Technology Review that the paper was rejected during the peer review process and would not be published. Harrisburg University confirmed this to Mic, stating, "The publication where it was scheduled to appear has since rejected the final version of the paper after a thorough peer-review process."
While this particular research project might be shelved, the idea of predictive policing is still alive and well. For years, technology companies and researchers have made promises of developing systems that would help police identify crimes before they happen. The promise of predictive policing is relatively simple and might even seem intuitive: feed previous crime data into an algorithm and it will spit out probabilities predicting where crime could happen next. The problem with it is the data that informs predictive policing programs is often based on existing bad behavior committed by law enforcement.
A study published last year by New York University School of Law and NYU’s AI Now Institute found that many predictive policing systems rely on "dirty data" based on flawed, racially-biased, and sometimes unlawful practices. For instance, in Chicago, the study found that a predictive system used criminal data that was collected during a period of time that the city's police department was under federal investigation for unlawful police practices. The demographics of people who the Department of Justice determined were victims of police bias were the same people targeted more frequently by the automated system. "You would necessarily be replicating the biased datasets from police records, which historically disproportionately included African Americans, and teaching the model based on those biased training data," Ferguson explains. Rather than actually predict where crime is most likely to occur, the system instead automates the discriminatory and abusive practices of a police force that suffers from implicit and explicit bias throughout its ranks.
The same concept of "dirty data" can apply to facial recognition technology. The Harrisburg University project is an extreme example, claiming to be able to predict a person's criminal tendencies based solely on a photo — a concept that seems at least distantly related to phrenology, a debunked and racist 19th century theory that claimed skull shapes can predict mental traits and behaviors. "It’s just stupid to think that a photograph or any biometric can predict future actions," Ferguson says. "Just plain stupid and a bit racist that our faces determine our actions."
But facial recognition is not foreign to police. Prior to recent reversals on the programs, companies like Amazon and IBM licensed facial recognition systems to local, state, and federal law enforcement agencies. These systems have long been criticized for racial bias. A study conducted by MIT Media Lab in 2019 found that Amazon's Rekognition technology regularly misidentified people with darker skin and would often mistake darker-skinned women for men. The ACLU produced similar findings in 2018 when it showed that Amazon's technology falsely matched 28 members of Congress, a disproportionate number of which were people of color, with criminal mugshots. Despite these troubling flaws that expose people of color to harsher policing and false accusations made against them, tech companies continued to provide the systems to law enforcement.
The effects of these AI systems that automate existing biases are starting to show up in policing practices. The New York Times recently highlighted the case of Robert Julian-Borchak Williams, a Black man in Farmington Hills, Michigan who was falsely accused of a crime based on facial recognition technology. Detroit police arrested Williams, held him in jail overnight, and accused him of shoplifting watches, based entirely on a facial recognition algorithm that falsely identified him. While Williams is believed to be the first known case of a citizen being wrongfully arrested because of the technology, it's unlikely he is actually the first to be subject to such unwarranted scrutiny.
The ACLU has warned that police in Florida have convicted people of crimes based largely on facial recognition systems and offered no recourse to challenge the technology's accuracy. There are likely many more like Williams out there who may simply not be aware of a flawed algorithm's role in their arrest. There will be many more if reckless research like the "predictive criminality" project conducted at Harrisburg University is allowed to continue and if law enforcement continues to rely on unproven and dirty data to drive their practices.