Scientists say your tweets could predict future coronavirus surges
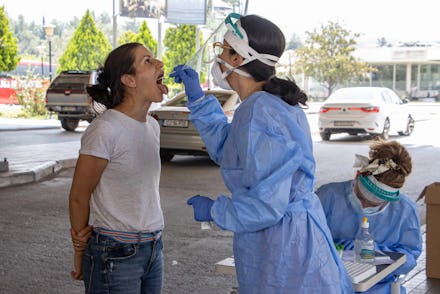
For much of the country, the first wave of coronavirus never truly went away. The spread seemed as though it was starting to slow, prompting public officials to re-open their economies and preemptively declare victory over the virus. In many cases, those same officials were slow to recognize when the disease was having a resurgence and failed to act quickly enough to stop it.
Now, scientists have a plan to take that decision making out of the hands of humans. In a paper published last week on arXiv.org, researchers laid the groundwork for a predictive model that could identify coronavirus outbreaks two weeks before they happen, allowing elected officials and public health experts to address the problem before it gets out of hand.
The algorithmic approach to identifying upticks in coronavirus cases doesn't rely on hospital capacity, positive test rates, or other standard indicators that aren’t able to predict the future. Instead, the scientists use real-time monitoring of search terms related to coronavirus on Google, posts about the virus and conditions on Twitter and other social media, mobility data gathered from smartphones, and a number of other digital markers that may suggest more people are experiencing the virus. According to researchers, coronavirus related searches on Twitter see a significant uptick two or three weeks in advance of increases in confirmed cases of the virus. Similar advanced warnings were provided by monitoring Google Trends.
The paper, which has not yet been peer-reviewed, suggests that the system could act as a sort of public health thermostat. If it senses cases going up, it can indicate to officials that action is needed to limit exposure by capping the capacity of certain businesses or restricting indoor activity and overall mobility until the spike is stopped. Likewise, if cases are on the decline and so are the early indicators that the algorithm monitors, it can lead officials to re-open more parts of society and the economy with a higher level of certainty that the public will be safe.
It's worth noting that some of the methods utilized by this modeling technique have been tested before, occasionally with less than stellar results. For example, Google made a significant to-do about using search terms to predict the severity of the flu season. Researchers at the company first floated the idea in 2008, and then Google made good on it by introducing Google Flu Trends in 2013. In its first flu season, it failed spectacularly. Researchers found that the system missed 2013's peak flu season by 140 percent. But that high profile failure hasn't stopped other researchers from trying similar methods of tapping into user search data to identify health trends. A team of researchers at Microsoft published a paper in 2016 that suggested Bing searches contained early indicators for diagnosis of pancreatic cancer. Another study, published last year, found that Google searches for health-related information often spike prior to a visit to the emergency room.
While the actual results of these data-based predictive systems may vary, the proposed coronavirus model certainly has potential. Identifying spikes before they happen will be increasingly important for communities to address the spread of the disease, and the proposed data-heavy method is relatively easy to set up and monitor. Scientists have been searching for early indicators that may provide warning signs before testing reveals an uptick, particularly because testing usually occurs only once a person is experiencing symptoms and may have already exposed others to the virus. Poop may serve as a useful warning sign, as the virus can potentially be identified in wastewater before people start experiencing symptoms.
Whether it’s human waste or an algorithm providing the warning sign, it'll be up to officials to actually act on it and on the general public to go along with rule changes. Without that cooperation, early warning signs will only serve as previews of upcoming catastrophes instead of avoidable dark fates.