Standardized tests are historically racist and classist — adding AI will just make it worse
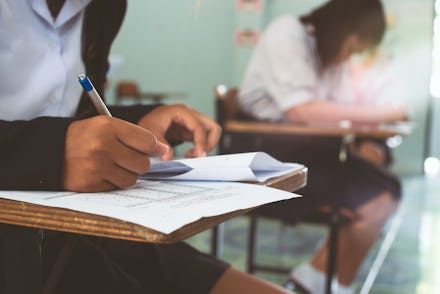
For years, standardized tests have been the barriers that students have to climb in order to advance academically. They are the ruler by which success is measured, despite being deeply flawed creations of a racist and classist system that has institutionalized biases against students of color. Now, according to a report from Vice, a number of states have turned over the grading process of exams and even essays to algorithms that display ongoing bias against certain demographic groups — further putting the people who are most in need of opportunity at a disadvantage.
According to Vice, there are now at least 21 states in the U.S. that rely on natural language processing (NLP) artificial intelligence systems, also known as automated essay scoring engines, to grade standardized tests. Three of those states claimed to also ensure every essay is graded by a person. For the remaining 18, just a small portion of essays, between five and 20 percent, are selected at random to have a human comb through in order to ensure the quality of the computer's grading process. That leaves the vast majority of students, who often have their access to higher education on the line, at the whim of an algorithm.
Troublingly, these automated graders suffer from built-in biases based on the way they are taught to look for mistakes and errors. Unlike human graders who are able to interpret information in front of them, particularly when given the subjective task of grading an essay, an algorithm only knows what to look for what it is trained to grade on. In most cases, that isn't the quality of writing or the concept that the writer puts to the page or how successfully they make an argument. Instead, the only thing that essay scoring engines are particularly tuned for is spelling and grammar checks. It measures metrics like sentence length and strength of vocabulary. The rest, the stuff that a human might be able to successfully parse, is lost in translation. Per Vice, essay-scoring engines are trained by feeding the algorithm sets of essays that have already been graded by a human. The machine processes those results, systematizing the process of figuring out what is considered to be a good essay and a bad essay. It then takes that information and applies it to a new essay, predicting what grade a human would give it based on the patterns that it has come to understand. While that may be cheaper and less time-consuming than having a person comb through every word of an essay, it also codes the biases of human graders into the automated system.
This is something that experts in artificial intelligence have been warning against for some time now. Laura Douglas, an AI researcher and CEO of myLevels, warned that when we teach machines on information that already contains our own human biases, the algorithm often has a habit of amplifying those biases rather than correcting for them. This has been an issue in a number of fields that have relied on algorithms for decision making. In 2015, Pro Publica highlighted how an automated system used to guide sentencing in criminal cases displayed racial bias by suggesting black defendants pose a higher risk of recidivism than they actually do while displaying an opposite bias for white defendants, predicting a far lower rate of recidivism. Likewise, an algorithm used by predictive crime tool PredPol had a tendency to disproportionately send police into minority neighborhoods regardless of actual crime stats in those areas, according to the Human Rights Data Analysis Group. Train machines with biased data and you will end up with biased results.
Essay grading is no exception to this rule. Because the companies that provide automated essay scoring engines are often protective of their algorithms and disclosing scores of tests requires the consent of test-takers, it can be hard to actually study the bias of these systems. However, the instances of research into automated grading show that the algorithms are leaving behind vulnerable students. Data published by the Educational Testing Service (ETS) to highlight the results of its E-rater grading engine found that it often gave higher grades to students from mainland China than humans would. The machine also under-scored African American students and, in some years, showed bias against Arabic, Spanish, and Hindi speakers.
As Vice reported, that bias can do a significant amount of damage to a student's grade — which can be essential to their opportunity to continue on to higher education. The essays graded by the E-rater system are scored on a scale of 0 to six. When the grades assigned by the machine were compared to those given by human graders, it was found that students from China were getting a boost of 1.3 points on average. Meanwhile, African Americans were downgraded by an average of 0.81 points.
Those scoring discrepancies go back to the bias built into the machines. When training the algorithm, essays that displayed a wide range of vocabulary, long sentences and correct spelling often performed well. In turn, that's what the machine looked for when grading a paper. The problem with that is that those metrics don't necessarily correlate to good writing or following instructions. Years ago, a group of students at MIT developed the Basic Automatic B.S. Essa Language (BABEL) Generator to put together what amount to complete nonsense sentences. However, while it reads as nothing more than gibberish to a human, a machine grader recognizes the use of big words, long sentences and proper spelling and grammar rather than the actual coherence of the essay. As a result, those complete nonsensical essays often score quite well when run through automated graders.
Students already face a considerable amount of human bias when going through the standardized testing process. The tests are far better at revealing gaps in opportunity than they are at showing gaps in actual skills. They also have historically favored white students by testing for information that is more often taught at predominantly white schools. A paper published in the Santa Clara Law Review also showed how bias can rear its head when determining what questions will be used on the test. The research highlighted instances of SAT test writers throwing out questions that were answered correctly by a higher percentage of black students than white students. Similarly, the testers chose to include a question that the vast majority of black students got wrong while a higher percentage of white students answered it correctly.
These types of tests have, from their inception, contained biases on the lines of race, class, and background. Those human tendencies have only been deeper ingrained into the algorithms that more and more states are counting on to do the grading of students for them. As a result the worst tendencies are only being exaggerated on tests that already put certain students at a disadvantage simply because of their race and economic factors that are beyond their control. This system is worsening inequalities that should be taken into account to provide additional opportunities, resulting in the people who need those chances the most being left behind.